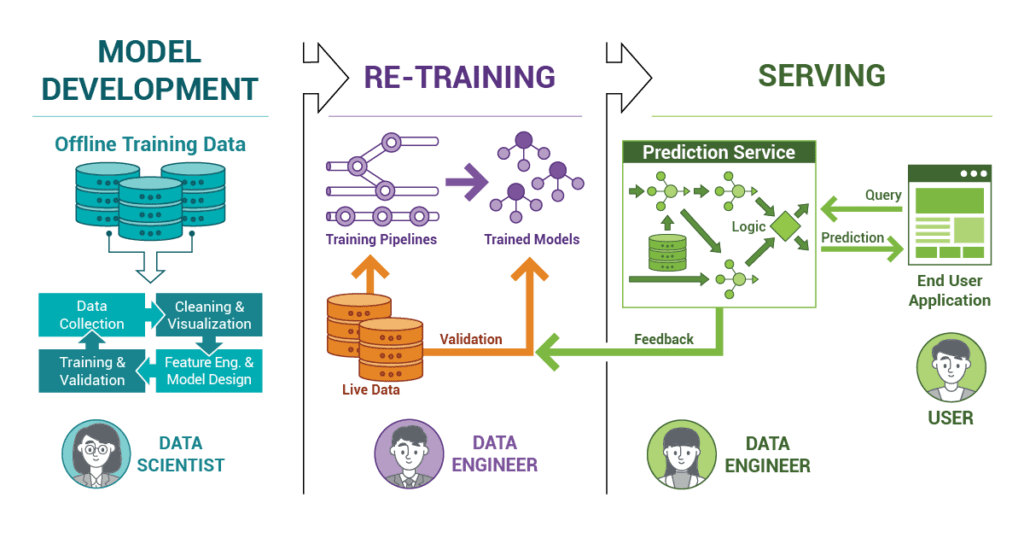
From Lab to Live: A Deep Dive into Machine Learning Model Deployment
An extensive guide to deploying machine learning models, exploring various deployment types, essential tools, and best practices for taking your models from development to production.
From Lab to Live: A Deep Dive into Machine Learning Model Deployment
Introduction
Developing a high-performing machine learning model is a significant achievement, but it's only half the battle. The true power of these models is unlocked when they are deployed into real-world applications, where they can generate predictions, automate tasks, and drive business value. This crucial step, known as model deployment, is often complex and multifaceted, requiring careful planning and execution.
This blog post will serve as your comprehensive guide to the world of machine learning model deployment. We'll move beyond the theoretical aspects of model building and delve into the practicalities of making your models accessible and impactful. We will explore various types of deployment, discuss essential tools and technologies, and outline the key considerations for a successful transition from the lab to a live environment. Get ready to navigate the landscape of ML deployment and learn how to bring your AI solutions to life!
1. Understanding the Model Deployment Landscape
Before diving into specific deployment types, it's crucial to understand the broader landscape. Model deployment is not a one-size-fits-all process. The optimal approach depends heavily on factors such as:
- Application Requirements: Is your application real-time or batch-oriented? What are the latency requirements? What is the expected traffic volume?
- Infrastructure Constraints: Do you need to deploy to the cloud, on-premises servers, edge devices, or a combination? What are your resource limitations (compute, memory, network)?
- Scalability Needs: Will your application need to scale to handle increasing data volumes or user traffic?
- Cost Considerations: What is your budget for deployment infrastructure and maintenance?
- Security and Compliance: What are the security and compliance requirements for your application and data?
- Team Expertise: What are the skills and expertise of your team in terms of DevOps, cloud technologies, and infrastructure management?
Answering these questions will help you narrow down the most suitable deployment strategies and tools for your specific use case.
2. Types of Machine Learning Model Deployment
Let's explore some of the most common and effective types of machine learning model deployment:
2.1. Cloud Deployment
Overview: Cloud deployment involves hosting your machine learning models and their serving infrastructure on cloud platforms like AWS, Google Cloud, or Azure. This is arguably the most popular and versatile deployment type.
Characteristics:
- Scalability and Elasticity: Cloud platforms offer immense scalability, allowing you to easily scale your resources up or down based on demand.
- Managed Services: Cloud providers offer managed services for model deployment, such as AWS SageMaker, Azure Machine Learning, and Google Cloud Vertex AI, which simplify many aspects of deployment, monitoring, and management.
- Global Reach: Deploy your models globally with ease, leveraging the worldwide infrastructure of cloud providers.
- Cost-Effective (Potentially): Pay-as-you-go pricing models can be cost-effective, especially for applications with variable traffic. However, costs can escalate if not managed properly.
- Ease of Management: Managed services reduce the operational burden of managing infrastructure, allowing teams to focus on model development and improvement.
Use Cases: Web applications, mobile apps, high-traffic APIs, applications requiring global accessibility, and scenarios where scalability and ease of management are paramount.
Tools & Technologies:
- Cloud Platforms: AWS (SageMaker, EC2, ECS, Lambda), Azure (Machine Learning, AKS, Azure Functions), Google Cloud (Vertex AI, GKE, Cloud Functions).
- Containerization: Docker, Kubernetes (Managed Kubernetes services like EKS, AKS, GKE).
- Serverless Functions: AWS Lambda, Azure Functions, Google Cloud Functions (for event-driven, scalable inference).
- Model Serving Frameworks: Cloud provider specific services, TensorFlow Serving, TorchServe, MLflow Serving (deployed on cloud infrastructure).
2.2. Edge Deployment
Overview: Edge deployment involves running machine learning models directly on edge devices, closer to the data source. Edge devices can be anything from smartphones and IoT sensors to industrial robots and autonomous vehicles.
Characteristics:
- Low Latency: Reduces latency significantly as data processing and inference happen locally, crucial for real-time applications.
- Offline Inference: Enables model inference even without a constant internet connection, vital for remote or disconnected environments.
- Reduced Bandwidth Consumption: Less data needs to be transmitted to the cloud, saving bandwidth and communication costs.
- Enhanced Privacy and Security: Data processing at the edge can enhance privacy by minimizing data transfer to central servers.
- Resource Constraints: Edge devices often have limited computational resources (CPU, memory, power), requiring model optimization and efficient deployment strategies.
Use Cases: Autonomous vehicles, industrial automation, smart cities, remote monitoring, applications with strict latency requirements, and scenarios with limited or unreliable network connectivity.
Tools & Technologies:
- Model Optimization Libraries: TensorFlow Lite, ONNX Runtime, Apache TVM (for model compression, quantization, and optimization for resource-constrained devices).
- Edge Computing Platforms: NVIDIA Jetson, Google Coral, Raspberry Pi.
- Mobile ML Frameworks: Core ML (iOS), TensorFlow Lite (Android).
- Edge Orchestration: KubeEdge, AWS IoT Greengrass (for managing and deploying models on fleets of edge devices).
2.3. Batch Deployment
Overview: Batch deployment is suitable for scenarios where predictions are needed for large datasets at once, and real-time or immediate responses are not required. Predictions are generated in batches, typically on a scheduled basis.
Characteristics:
- High Throughput: Efficient for processing large volumes of data in a non-real-time manner.
- Cost-Effective for Large Datasets: Can be more cost-effective than real-time deployment for processing massive datasets as resources can be utilized optimally during batch processing windows.
- Simpler Infrastructure: Often requires less complex and less demanding infrastructure compared to real-time deployments.
- Delayed Results: Predictions are not immediately available, which is acceptable for applications where immediate responses are not critical.
- Scheduled Execution: Batch jobs are typically scheduled to run periodically (e.g., daily, hourly, weekly).
Use Cases: Fraud detection (daily or nightly batch processing), customer churn prediction, marketing campaign targeting, risk assessment, report generation, and scenarios where insights are needed periodically rather than instantaneously.
Tools & Technologies:
- Batch Processing Frameworks: Apache Spark, Hadoop MapReduce, Apache Beam (for distributed data processing).
- Workflow Orchestration: Apache Airflow, Prefect, Dagster (for scheduling and managing batch jobs).
- Databases and Data Warehouses: Cloud data warehouses (Snowflake, BigQuery, Amazon Redshift) for storing and processing large datasets.
- Cloud Compute Services: AWS EC2, Azure Virtual Machines, Google Compute Engine (for running batch processing jobs).
2.4. Real-time (Online) Deployment
Overview: Real-time deployment, also known as online deployment or serving, provides immediate predictions on demand. Models are deployed as services that respond to individual requests with low latency.
Characteristics:
- Low Latency Inference: Designed for applications requiring immediate responses, typically within milliseconds or seconds.
- High Availability: Requires robust infrastructure to ensure continuous availability and handle concurrent requests.
- Scalability for Request Volume: Needs to scale to handle varying levels of incoming prediction requests.
- Stateful or Stateless: Can be implemented as stateless services (easier to scale) or stateful services (for models requiring persistent state).
- More Complex Infrastructure: Often involves more complex infrastructure setup and management compared to batch deployment.
Use Cases: Recommendation systems, online fraud detection (transaction-level), personalized search, real-time language translation, chatbots, applications demanding immediate feedback and interaction.
Tools & Technologies:
- Model Serving Frameworks: TensorFlow Serving, TorchServe, MLflow Serving, Triton Inference Server, Seldon Core, KFServing (optimized for low-latency, high-throughput serving).
- API Gateways: Kong, NGINX (for request routing, load balancing, and security).
- Container Orchestration: Kubernetes (for managing and scaling real-time serving infrastructure).
- Caching Mechanisms: Redis, Memcached (to reduce latency for frequently requested predictions).
- Cloud Load Balancers: Cloud provider load balancers (ELB, ALB, Google Cloud Load Balancing, Azure Load Balancer) for distributing traffic.
2.5. Serverless Deployment
Overview: Serverless deployment leverages serverless computing platforms to deploy and run machine learning models. Serverless functions are event-driven, automatically scale, and you only pay for the compute time consumed during execution.
Characteristics:
- Automatic Scaling: Serverless platforms automatically scale based on incoming requests, handling traffic spikes without manual intervention.
- Pay-per-Execution Pricing: Cost-effective for applications with infrequent or variable usage, as you only pay for the actual compute time used.
- Simplified Operations: Reduces operational overhead as the cloud provider manages the underlying infrastructure (servers, scaling, patching).
- Event-Driven Architecture: Well-suited for event-driven applications where predictions are triggered by specific events (e.g., new data arrival, user actions).
- Cold Starts: Potential "cold start" latency when a serverless function is invoked after a period of inactivity.
Use Cases: Microservices, event-driven applications, APIs with variable traffic patterns, background processing tasks, and scenarios where operational simplicity and cost efficiency for intermittent workloads are priorities.
Tools & Technologies:
- Serverless Platforms: AWS Lambda, Azure Functions, Google Cloud Functions.
- Container-based Serverless: AWS Fargate, Google Cloud Run, Azure Container Instances (for running containerized models in a serverless manner).
- Serverless Model Serving Frameworks: Frameworks optimized for serverless environments or adaptable to serverless deployment.
3. Essential Tools and Technologies for ML Model Deployment
The success of your model deployment relies heavily on the right tools and technologies. Here's an overview of key categories and examples:
3.1. Containerization and Orchestration
Containers (Docker, Podman): Containers package your model, its dependencies, and runtime environment into a portable and isolated unit. Docker is the most popular containerization technology.
Orchestration (Kubernetes, Docker Swarm): Kubernetes is the leading container orchestration platform. It automates the deployment, scaling, and management of containerized applications. Kubernetes is crucial for managing complex deployments, especially in cloud and real-time scenarios. Docker Swarm is a simpler alternative for smaller deployments.
Benefits:
- Consistency: Ensures consistent execution environments across development, testing, and production.
- Portability: Containers can run on any platform supporting container runtimes.
- Scalability and Resilience: Kubernetes enables horizontal scaling and automated recovery from failures.
- Resource Efficiency: Containers share the host OS kernel, making them more resource-efficient than virtual machines.
3.2. Model Serving Frameworks
Model serving frameworks are specialized tools designed to efficiently serve machine learning models in production.
Examples:
- TensorFlow Serving: A high-performance serving system for TensorFlow models, optimized for speed and scalability.
- TorchServe: A flexible and easy-to-use serving framework for PyTorch
- TorchServe: A flexible and easy-to-use serving framework for PyTorch models, emphasizing simplicity and ease of deployment.
- MLflow Serving: Part of the MLflow platform, it allows serving models from various ML frameworks (including scikit-learn, TensorFlow, PyTorch) and integrates well with MLflow's model registry.
- NVIDIA Triton Inference Server (formerly TensorRT Inference Server): A high-performance inference server optimized for NVIDIA GPUs, supporting multiple frameworks and designed for high throughput and low latency.
- Seldon Core: An open-source platform for deploying, managing, and monitoring machine learning models on Kubernetes. It supports advanced deployment patterns like canary deployments and A/B testing.
- KFServing (now part of Kubeflow): Another Kubernetes-based platform for serving ML models, focused on serverless inference and integration with Kubeflow's MLOps ecosystem.
Benefits:
- Optimized for Inference: Frameworks are specifically built for efficient model inference, handling requests, and managing model versions.
- Abstraction: They abstract away many low-level details of serving, allowing data scientists and ML engineers to focus on model deployment logic.
- Scalability and Performance: Designed for scaling to handle production traffic and delivering low-latency predictions.
- Integration with Ecosystems: Often integrate with broader ML ecosystems (like MLflow, Kubeflow) and cloud platforms.
3.3. Infrastructure as Code (IaC) and Automation
![Infrastructure as Code(https://miro.medium.com/v2/resize:1400/1*Sl27vO7NlB6oaZ0xG894LQ.png)
IaC Tools (Terraform, AWS CloudFormation, Azure Resource Manager, Google Cloud Deployment Manager): IaC allows you to define and manage your deployment infrastructure using code. This enables automation, version control, and repeatability of infrastructure setup.
Automation Tools (Ansible, Chef, Puppet): These tools automate configuration management, software installation, and deployment tasks, reducing manual effort and ensuring consistency.
Benefits:
- Automation: Automates infrastructure provisioning, configuration, and deployment processes, reducing manual errors and speeding up deployments.
- Version Control: Infrastructure code can be version-controlled, allowing you to track changes and roll back to previous configurations.
- Repeatability and Consistency: Ensures consistent infrastructure deployments across different environments (development, staging, production).
- Improved Collaboration: Code-based infrastructure facilitates collaboration between development and operations teams.
3.4. Monitoring and Logging Tools
Monitoring Platforms (Prometheus, Grafana, Datadog, New Relic, CloudWatch, Azure Monitor, Google Cloud Monitoring): These platforms provide comprehensive monitoring of your deployed models and infrastructure, tracking metrics like latency, throughput, error rates, resource utilization, and model performance.
Logging Tools (ELK Stack (Elasticsearch, Logstash, Kibana), Splunk, Fluentd): Logging tools collect, aggregate, and analyze logs from your deployment environment, aiding in debugging, troubleshooting, and auditing.
Benefits:
- Performance Monitoring: Track model performance in production, identify bottlenecks, and ensure service level agreements (SLAs) are met.
- Model Drift Detection: Monitor for data drift and concept drift, which can degrade model accuracy over time.
- Error Detection and Debugging: Quickly identify and diagnose errors in your deployment environment.
- Alerting and Notifications: Set up alerts to be notified of critical issues, performance degradation, or anomalies.
4. Building Robust Deployment Pipelines and Embracing MLOps
To achieve reliable, scalable, and maintainable model deployments, it's essential to establish automated deployment pipelines and adopt MLOps (Machine Learning Operations) practices.
Deployment Pipelines:
A deployment pipeline automates the process of taking a trained model and deploying it into a production environment. A typical pipeline includes stages like:
- Model Packaging: Packaging the trained model and its dependencies into a deployable artifact (e.g., container image, model archive).
- Testing and Validation: Automated testing of the deployed model in a staging environment, including integration tests, performance tests, and security scans.
- Deployment to Target Environment: Deploying the validated model to the production environment using chosen deployment strategies (e.g., blue/green, canary).
- Monitoring and Rollback: Setting up monitoring and automated rollback mechanisms in case of deployment failures or performance issues.
MLOps Principles:
MLOps is a set of practices that aims to bring DevOps principles to machine learning. It focuses on streamlining the entire machine learning lifecycle, from model development to deployment, monitoring, and governance. Key MLOps principles include:
- Automation: Automating as many steps as possible in the ML lifecycle, including data validation, model training, testing, deployment, and monitoring.
- Continuous Integration and Continuous Delivery (CI/CD) for ML: Implementing CI/CD pipelines for automated model building, testing, and deployment.
- Model Versioning and Reproducibility: Tracking model versions, datasets, code, and configurations to ensure reproducibility and facilitate model management.
- Monitoring and Feedback Loops: Continuously monitoring model performance in production and using feedback to improve models and deployment processes.
- Collaboration and Communication: Fostering collaboration between data scientists, ML engineers, DevOps engineers, and business stakeholders.
Benefits of MLOps:
- Faster Time to Market: Automated pipelines and streamlined processes accelerate the deployment of ML models.
- Improved Reliability and Stability: Automated testing, monitoring, and rollback mechanisms enhance the reliability and stability of deployed models.
- Increased Scalability and Efficiency: MLOps practices enable efficient scaling of ML infrastructure and operations.
- Better Governance and Compliance: Versioning, audit trails, and monitoring improve governance and compliance.
- Reduced Operational Costs: Automation and efficient resource utilization can reduce operational costs.
5. Key Considerations for Successful Model Deployment
Beyond choosing the right deployment type and tools, several key considerations are crucial for successful model deployment:
5.1. Security
Security is paramount in model deployment. Consider these aspects:
- Model Security: Protect your trained models from unauthorized access and modification. Implement access controls and encryption.
- Data Security: Ensure the security and privacy of data used for inference and model training. Comply with data privacy regulations.
- API Security: Secure your model serving APIs using authentication, authorization, and rate limiting to prevent abuse and unauthorized access.
- Infrastructure Security: Secure your deployment infrastructure (servers, containers, networks) by following security best practices and regularly patching vulnerabilities.
- Vulnerability Scanning: Implement automated vulnerability scanning of your container images and infrastructure components.
5.2. Scalability and Performance
Ensure your deployment can handle current and future demands:
- Horizontal Scaling: Design your deployment to scale horizontally by adding more instances to handle increased traffic. Kubernetes is excellent for this.
- Load Balancing: Use load balancers to distribute traffic evenly across model serving instances.
- Caching: Implement caching mechanisms to reduce latency and improve performance for frequently requested predictions.
- Performance Optimization: Optimize your models for inference speed and resource efficiency. Techniques include model quantization, pruning, and efficient model architectures.
- Resource Monitoring and Autoscaling: Monitor resource utilization and set up autoscaling to automatically adjust resources based on demand.
5.3. Monitoring and Management
Continuous monitoring is essential for maintaining model performance and identifying issues:
- Comprehensive Monitoring: Monitor key metrics like latency, throughput, error rates, resource utilization, model accuracy, and data drift.
- Alerting and Notifications: Set up alerts for critical metrics and anomalies to be notified of potential problems.
- Logging and Tracing: Implement robust logging and tracing to facilitate debugging and troubleshooting.
- Model Drift Detection: Actively monitor for data drift and concept drift and have strategies to retrain or update models when drift is detected.
- Centralized Dashboards: Use centralized dashboards to visualize monitoring data and get a holistic view of your deployment health.
5.4. Cost Optimization
Cloud deployments can become costly. Implement cost optimization strategies:
- Right-Sizing Resources: Choose appropriately sized instances and resources for your workload. Avoid over-provisioning.
- Autoscaling and Serverless: Leverage autoscaling and serverless functions to only pay for resources when they are actually used.
- Reserved Instances and Spot Instances (Cloud): Utilize reserved instances or spot instances for cost savings on cloud compute resources where applicable.
- Storage Optimization: Optimize data storage costs by using appropriate storage tiers and lifecycle policies.
- Monitoring Cost: Continuously monitor cloud spending and identify areas for cost reduction.
Conclusion
Deploying machine learning models is a critical step in realizing their value. This post has explored the diverse landscape of model deployment, from cloud and edge to batch, real-time, and serverless options. We've also delved into essential tools, technologies, and MLOps practices that are crucial for building robust and scalable deployments.
Choosing the right deployment strategy depends on your specific application requirements, infrastructure constraints, and business goals. By carefully considering these factors and embracing MLOps principles, you can successfully transition your models from the lab to live environments, unlocking their full potential and driving impactful results.
The journey of model deployment is continuously evolving, with new tools and techniques emerging regularly. Stay curious, keep learning, and experiment with different approaches to find the best solutions for your unique challenges.
If you're navigating the complexities of ML model deployment and want to discuss specific scenarios or challenges, let's connect! ( ^-^)**(^0^ )
Thank you for exploring the world of Machine Learning Model Deployment with me! Your questions and insights are always welcome. ╰(°▽°)╯